Research Framework
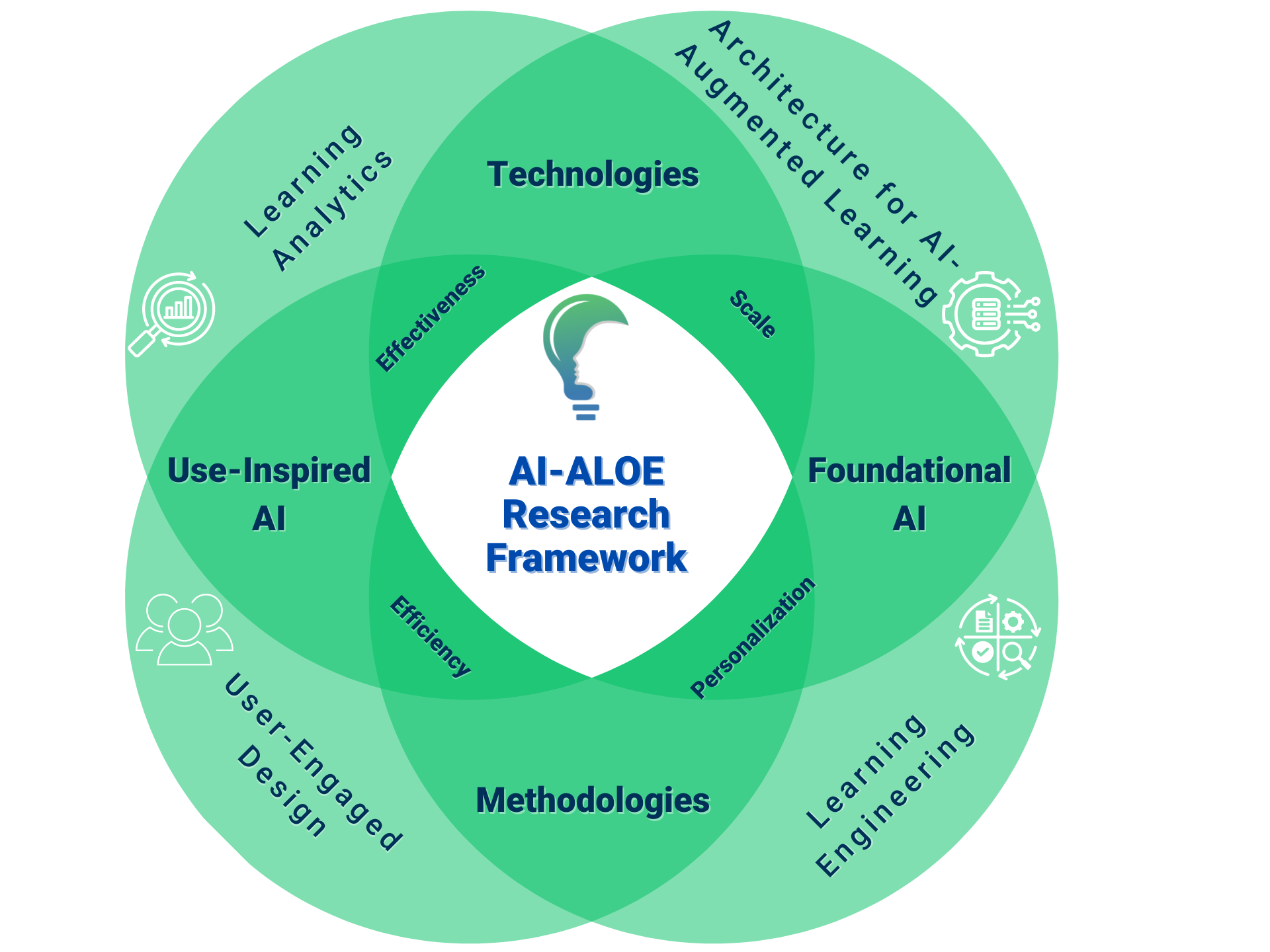
Research Thrusts
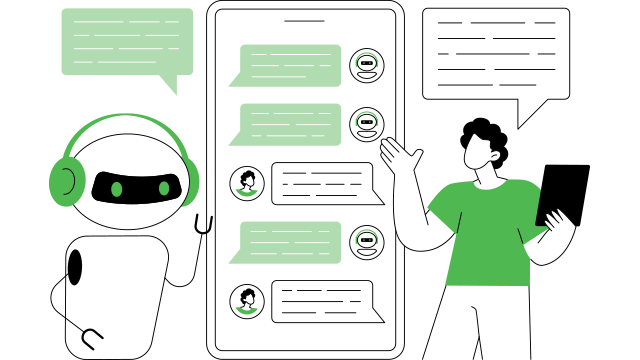
Thrust 1: AI-Assistants for Learning and Teaching
AI-ALOE develops AI learning assistants such as conversational textbooks and interactive videos to enhance cognitive engagement, as well as social assistants to improve teacher-learner and learner-learner interactions. It also develops AI teaching assistants to offload some of teachers’ work, for example, through automated assessment and automated question answering.
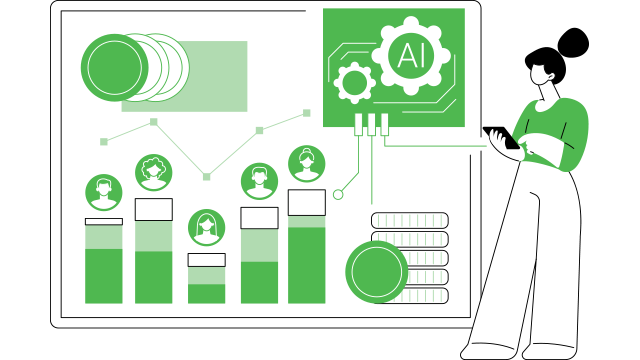
Thrust 2: Personalization of Learning at Scale
AI-ALOE develops AI interactive environments for personalization of learning at scale in the context of ill-defined problems and self-directed learning. This entails the development of new analytical techniques that can monitor and analyze the behavior of individual learners as well as new methods of coaching that can nudge learners toward more productive behaviors.
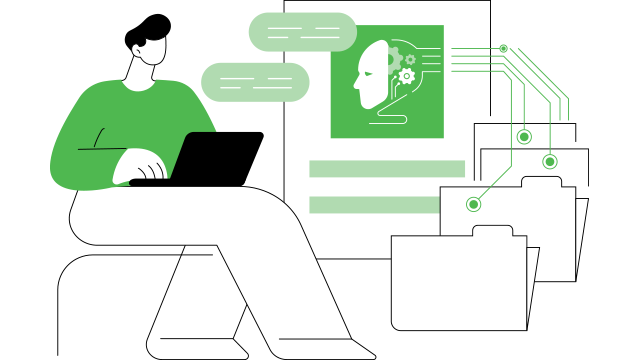
Thrust 3: Human-AI Interaction
AI-ALOE develops new AI models and techniques for (1) Self-explanation (an agent’s ability to explain its reasoning), (2) Information visualization (the ability to make data comprehensible to humans), (3) Machine teaching (the ability to interactively teach an AI agent), and (4) Mutual theory of mind (the ability of humans and AI agents to ascribe mental states to each other).
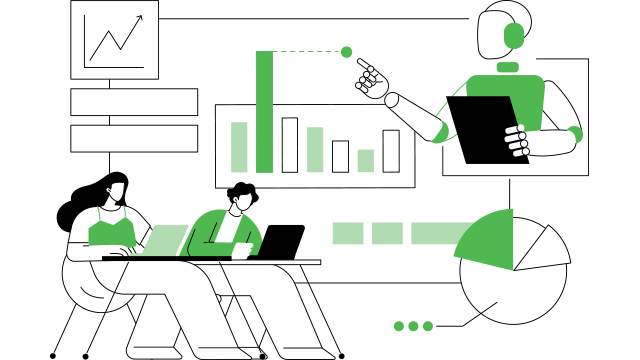
Thrust 4: Architecture for Learning
AI-ALOE is developing an Architecture for AI-Augmented Adult Learning (A4L). The A4L technology and data architecture enables deployment and evaluation of the AI assistants for learning and teaching in real educational contexts, collection and analysis of large-scale data on learning, and personalization of learning at scale. It also addresses the issues of data privacy, data security, and data sharing.
Research Design
The research design in our work derives from the theoretical foundation of feedback loops in human learning. Although some learners are very good at self-directed learning, most learners need customized guidance and support to acquire the required knowledge, skills, and dispositions. Effective guided learning engages positive, data-driven feedback loops, such as a teacher-centered loop and a learner-centered loop.
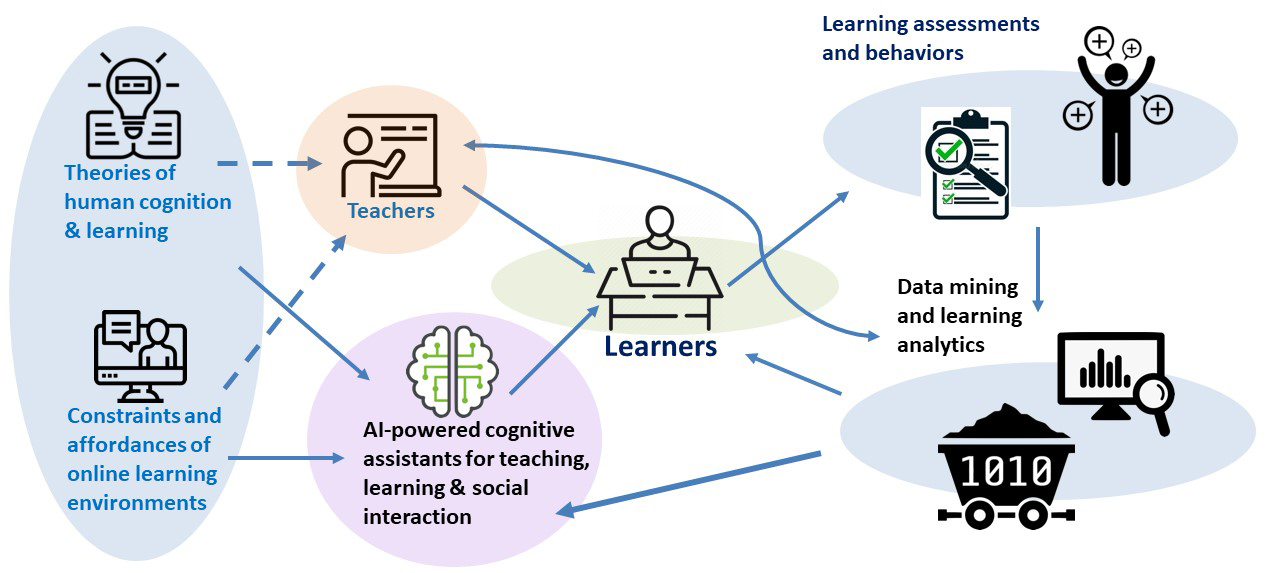