The SMART System and Concept Learning
Min Kyu Kim, Georgia State University
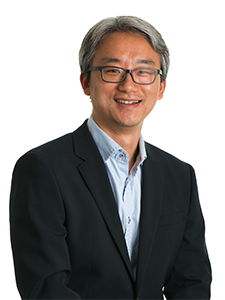
Min Kyu Kim
Min Kyu Kim is an associate professor in the College of Education and Human Development at Georgia State University. Kim received his Ph.D. from the Learning, Design, and Technology program at the University of Georgia in May 2012. He worked as a postdoctoral fellow at the University of Southern California (2012-2014) and The Ohio State University (2014-2016). His research pursues innovative studies that advance our understanding of how people learn and how to assess and foster transformative learning, especially in technology-rich learning environments. More specifically, Kim’s program of research focuses on designing and developing learning technologies that enable learners to receive personalized support, while also helping educators adapt problem-centered pedagogies by providing insights into individual students’ learning progress. He is dedicated to exploring AI techniques to collect, externally represent, and diagnose learner characteristics such as cognition, motivation, and emotion. In this effort, he founded the AI2 Research Laboratory that builds on theories of learning and instruction to create innovative learning environments that maximize learner capacity to achieve learning goals. The laboratory fosters interdisciplinary and cross-institutional collaborations, uniting experts in learning sciences, computer sciences, STEM educators, and literacy researchers from multiple institutions.
I’ve developed an AI technology known as the Student Mental Model Analyzer for Research and Teaching (SMART) system. SMART is a web-based platform where students engage with learning material. They summarize what they’ve read or describe their understanding of problem scenarios. SMART uses AI to analyze language data and concept maps, helping learners grasp concepts and assess their understanding. This system is particularly beneficial for adult learners engaged in concept learning, a process that builds a strong understanding of STEM content through reading or online video lectures. Concept learning, a key aspect of cognitive presence, involves constructing meaning through reflection, discourse, and critical thinking. AI applications like SMART support self-
paced concept learning, foster positive perceptions of AI in education, and even impact learner performance in other tasks. Over the past two years, I’ve implemented SMART in English and Biology classes at TCSG Colleges, benefiting around 400 adult learners. We hypothesized that SMART’s AI-based formative assessment and feedback would engage adult learners in concept learning and deepen their understanding. Additionally, we explored whether learners’ micro-level concept learning on SMART could enhance their transition to more complex STEM assignments. To address these questions, we gathered data from various sources, including interviews, discussions, surveys, and classroom scores. Analysis of this data revealed that adult learners effectively
engaged with SMART, constructing appropriate mental models of the materials. Their concept learning positively influenced motivation and classroom performance, indicating that SMART’s personalized support aided learners in reflection, regulation, and advancement of concept learning. SMART’s insights also supported instructors in understanding individual learner progress and refining their instruction.
Our work doesn’t stop here. We’re currently developing data visualization tools for SMART, allowing learners to assess their learning patterns and performance. This feature, available in Fall 2023, empowers learners to review their behavior and performance on SMART, while instructors gain insights to enhance online class management. We’re integrating ChatGPT with SMART, leveraging autonomous ChatGPT techniques to automate expert model generation for efficient text analysis. This project involves generating explainable AI feedback messages and personalized feedback tailored to individual learners. By incorporating generative AI, learners can engage with diverse writing examples and integrate them into their knowledge models. Through our research journey, we’ve explored SMART in two TCSG classes, conducting micro-level field tests that assess task performance and meso-level impacts on learner engagement and performance. As we move into Year 3, our focus shifts to concept learning in occupational skills and workforce development for adult learners in online reskilling and upskilling programs. We’ll employ Randomized Controlled Trials (RCTs) and quasi-experiment designs to examine how personalized learning at different levels impacts learners, considering micro, meso, and macro contexts. This research aims to uncover the intricate dynamics between learners’ constraints, experiences, and online learning with AI tools.