Visual Analytics to Understand, Personalize, and Trust AI in Adult Education
Alex Endert, Georgia Tech
Alex Endert is an associate professor in the School of Interactive Computing at the Georgia Institute of Technology. He directs the Visual Analytics Lab, which focuses on designing and studying how interactive visual tools assist individuals in comprehending data and AI. The lab frequently tests these advancements across various domains, including intelligence analysis, cybersecurity, learning, decision-making, manufacturing safety, among others. Generous support for their work comes from sponsors including NSF, DOD, DHS, DARPA, DOE, and industry partners.
Endert received his Ph.D. in Computer Science from Virginia Tech in 2012. In 2013, his work on Semantic Interaction was awarded the IEEE VGTC VPG Pioneers Group Doctoral Dissertation Award, and the Virginia Tech Computer Science Best Dissertation Award. In 2018, he received a CAREER award from the National Science Foundation for his work on
visual analytics by demonstration.
Our research in AI-ALOE aims to enable individuals involved in education to better interpret, understand, and monitor the use of AI for various tasks. The goal is to support three primary user groups involved in AI-ALOE: teachers, learners, and AI researchers who develop the AI agents for various purposes. Each user group faces specific challenges as their tasks and goals differ. For example, through focus groups and iterative design practices, we have discovered that teachers are primarily interested in understanding how learners utilize the AI agents in the context of the learning goals and pedagogical structure they envision. We have developed a visualization (called VisTA) to allow teachers to track how students utilize intelligent tutors to learn specific skills. Our findings indicate that beyond simple tracking and monitoring performance, typically resulting in assigning grades, teachers are more interested in understanding learning behavior. For example, visually exploring the data in aggregate reveals gaps in knowledge across a group of learners, leading to adjustments in materials or lessons to ensure students better understand the material.
Our research has also enabled AI developers to test, improve, and ultimately calibrate their trust and confidence in specific AI agents prior to deployment. Specifically, we have developed visual analytic tools to illuminate errors in how LLMs auto-grade text summaries written by students. These tools (iScore and KnowledgeVis, see figures below) have revealed biases and other linguistic errors, resulting in improvements in the design of LLMs before deployment. Capabilities like this aid in ensuring the ethical deployment and use of AIs for educational purposes in AI-ALOE.
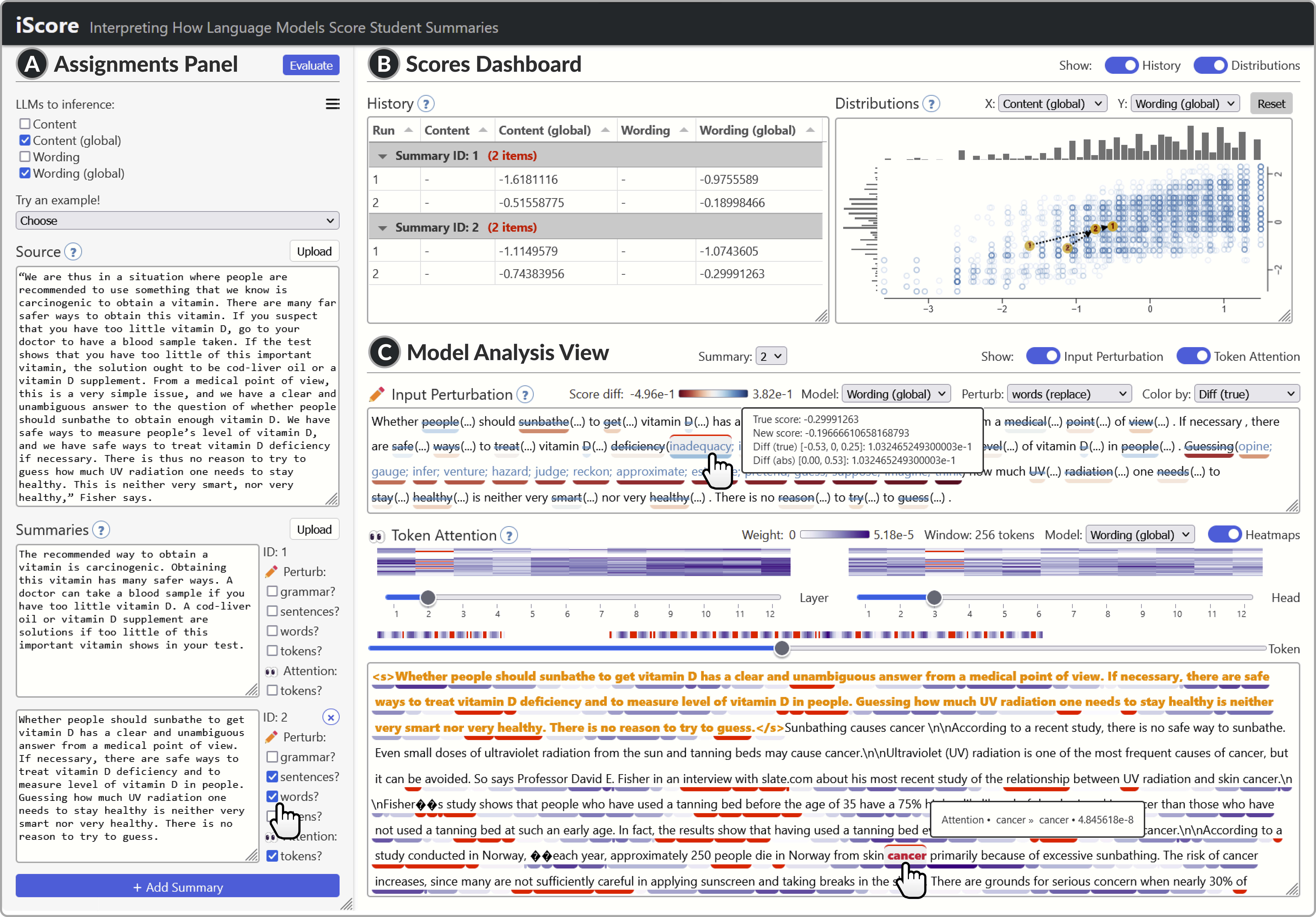
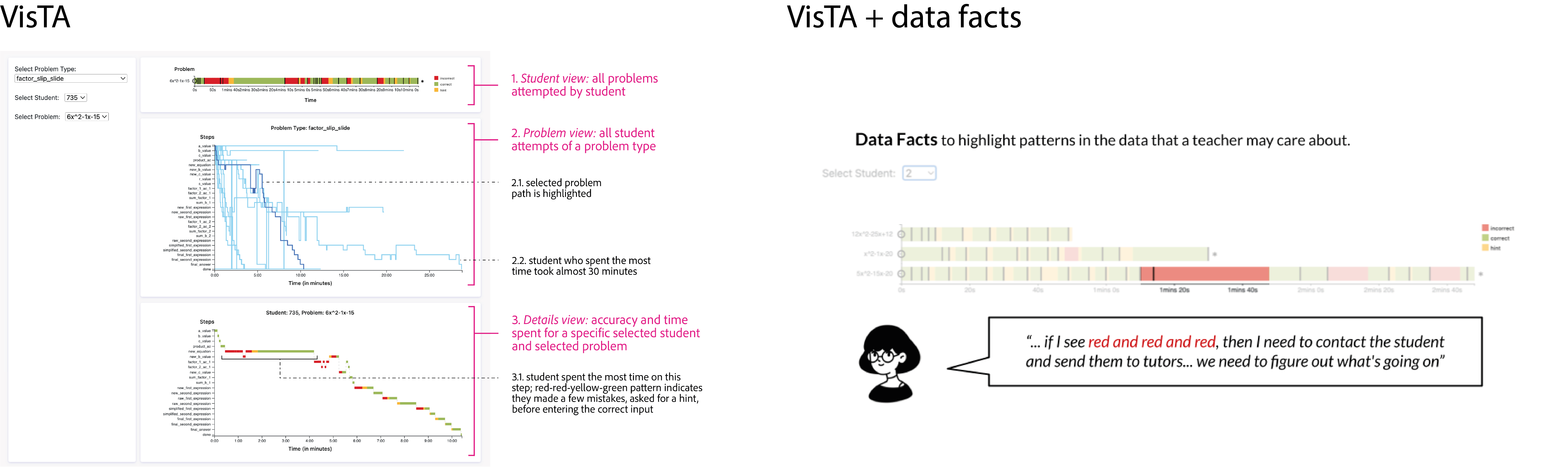